An Economists’ Take
In June, we had the opportunity to hear Professor Ajay Agrawal speak on the topic of AI. This was via Digi’s Global IoT Conference (see our earlier post for a larger event summary). Agrawal is a Professor of Strategic Management and Peter Munk Professor of Entrepreneurship at the Rotman School of Management for University of Toronto. In addition, he’s the co-founder of The Next 36, Kindred, and the Creative Destruction Lab, all organizations with an AI and robotics emphasis.
With a session themed around his research and book, Prediction machines: the simple economics of artificial intelligence, Agrawal broke down examples of where AI is bringing efficiency and impact. We have all witnessed examples of Prediction in action through AI – think, Google’s Smart Reply. Smart Reply provides three templated email replies based on senders’ email content. It allows Gmail users to select a response for simplification and, of course, time savings.
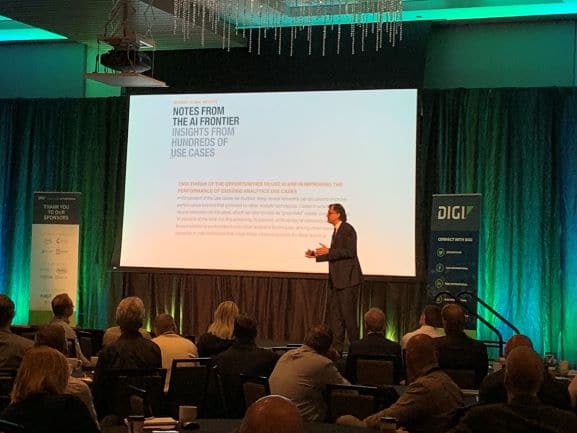
Decision-Making Workflow
More interestingly than examples of artificial intelligence in our everyday, though, was Agrawal’s insights on where AI isn’t yet as impactful. Through a dissection of the decision-making process, Agrawal offered a unique perspective where AI is not an all-encompassing replacement to human activities. With an emphasis on the 7-1 diagram exhibited below, he discussed the Inputs for coming to a conclusion. As explained in Ajay Agrawal’s book Prediction machines, which is co-authored by Joshua Gans and Avi Goldfarb (2018),
When someone (or something) makes a decision, they take input data from the world that enables a prediction. That prediction is possible because training occurred about relationships between different types of data and which data is most closely associated with a situation. Combining the prediction with judgment on what matters, the decision maker can then choose an action. The action leads to an outcome (which has an associated reward of payoff). The outcome is a consequence of the decision. It is needed to provide a complete picture. The outcome may also provide feedback to help improve the next prediction.
(p. 74)
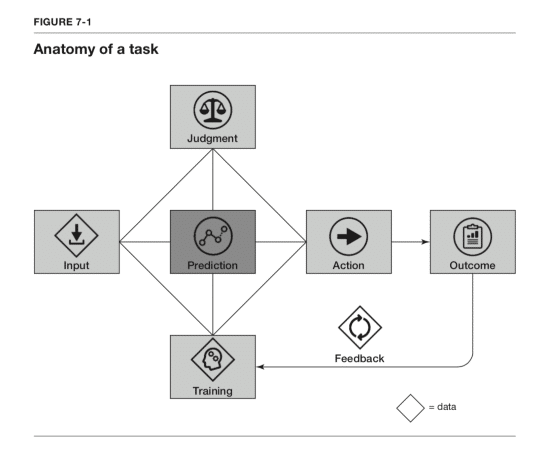
To summarize, with prediction being utilization of the information we have to generate information that we don’t, prediction machines (aka AI) have the most pressing impact at a decision level. However, as described in the above process flow, there are six additional steps in decision-making progression. These other steps are where human activities are still necessary.
With further conclusions as captured in the book,
But a key point is that, while prediction is a key component of any decision, it is not the only component. The other elements of a decision – judgment, data, and action – remain, for now, firmly in the realm of humans. They are complements to prediction, meaning they increase in value as prediction becomes cheap.
(Agrawal, Gans, and Goldfarb, 2018, p. 76)
Essentially, while the value placed on substitutes to prediction – such as human prediction – will decrease, the complementary values for the human additions to other decision-making steps – such as data collection – increase. This facet is important. While we can acknowledge that AI has made its mark in providing cheaper and faster predictions than humans, we must also acknowledge that they currently are only a slice of the process.
According to these authors with great AI expertise, judgment in particular is a human skill that will gain more importance. This is because the ability to decipher correct and incorrect decisions (and therefore, perceived rewards and mistakes) continues to be uniquely human.
Beyond Prediction
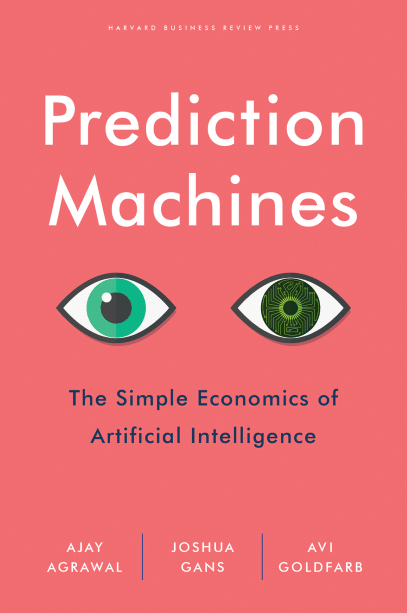
Given that our article focuses on insights as shared directly at the Conference, we wish to emphasize this is only a fragment of the topics provided in their book. For a more in-depth analysis, including in the area of societal influence, check out this book.
In addition, further articles and profiles for Agrawal, Gans, and Goldfarb can be found at the customized Prediction Machines site.
AI and Louisville
In addition, on a local level, we feel compelled to share about recent news regarding Microsoft and Louisville, KY. Coincidentally, shortly after hearing Agrawal speak, Microsoft announced they will open their first regional hub for AI in Louisville.
Coined the “AI Innovation Digital Alliance,” it will focus on research, training, and AI exploration, specifically related to IoT. As reported, Microsoft selected Louisville because of its digital inclusion efforts and the expected impact of automation on the city’s manufacturing and healthcare sectors. Along with equity in economic growth, a primary alliance goal is assisting workforce adaptions to the scale of automation.
Reference
Agrawal, A., Gans, J., and Goldfarb, A. (2018). Prediction machines: the simple economics of artificial intelligence. Boston, MA: Harvard Business Review Press.